Diving into the concept of measurement
By Richard Seiersen: Risk Management Author, Serial CISO, CEO Soluble
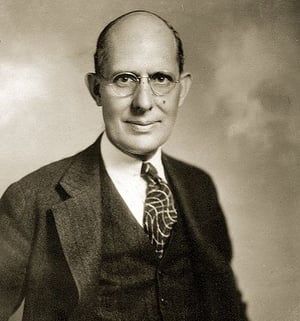
My favorite metrics quote is, “A problem well defined is a problem half solved,” by Charles Kettering. Kettering was an industrious guy responsible for 186 patents, including but not limited to the electric start motor, leaded gasoline, and freon.
His quote shows up in How To Measure Anything In Cybersecurity Risk during our discussion about the object of measurement. It also showed up in last week’s article when I said our “object” is, “Keeping the likelihood and impact of third party driven breach within risk tolerance given possible third party gains.”
You Can’t Predict That!
Some of you may disagree with my “object of measurement” by saying, “we lack the data, and even if we had it, we couldn’t predict that!” This is where my second favorite metrics quote comes in: “Although this may seem a paradox, all exact science is based on the idea of approximation. If a man tells you he knows a thing exactly, then you can be safe in inferring that you are speaking to an inexact man.”
Bertrand Russel said that. He was the 3rd Earl of Russell, a Nobel Laureate, and led the “Revolt Against Idealism.” He was pretty much a genius’ genius and a skeptics’ skeptic. The above quote cleverly states his perspective on “the concept of measurement.” It’s a point of view on measurement that most all other scientists would agree with.
The Concept Of Measurement
When you know absolutely nothing, a little data can go a long way. Imagine it’s your first day on the job as the first CISO of NewCo. NewCo is a fast moving software company. It’s just shy of one thousand people and predicted to double in size over the next two years. In your first meeting you find out that NewCo persist several billion records of customer data in one or more SaaS provider systems. (NewCo is cloud native.)
Do you have enough data to make a decision? Yes! And that decision is, “tell me more!” Let’s say that the next thing you learn is, “We have $15M in cyber insurance.” Now I present a trick question: “Do you have enough data to make a decision about changing your cyber insurance?”
The real question is, “how much data do I need, and at what cost, to make that decision?” If my standard for decision making is “I need perfect data or I won’t decide”, then not only am I in the wrong business, but Bertrand Russell won’t let me into his science club!
Continuing our story, let’s say it’s $60K for $10M more insurance or $110K for $20M more. Along the way you also find out that you have a five person team of junior security practitioners, a bug bounty program, and not much else. Oh, and you have been given a $250K budget for now.
This ends your first day as the newly minted CISO of NewCo. And what have you done? You have profoundly reduced your uncertainty with a little data. This is what the concept of measurement is all about. So, what’s next?
Risky Decisions - Defining Terms
I have been using the “decision” word a lot. I probably should define it. A decision is “an irrevocable allocation of resources.” At least that is how the grandfather of decision science Prof Ron Howard puts it. So, it’s not a mental exercise but a transaction of some sort.
Based on the data you have accrued in 24 hours it seems like NewCo may be at risk and a decision needs to be made. When I say risk I mean it like Sam Savage does. Sam is an itinerant professor at Stanford, founder of Probability Management and author of the great book The Flaw Of Averages. In it he states, “Risk is in the eye of the beholder.” In short, there is no purely objective standard for risk. It’s relative.
Your decision may be, “Do I buy more insurance now (which will take a few weeks to become active) or should I do an assessment using my limited resources and decide later?” It’s a risky decision. Wait too long to act and something bad could happen. Spend frivolously and you could delay betterments that could protect you.
When you think like this, as I am sure you often do, you are doing something very important. You are modeling risk! You indeed have a model, probably several, they are just floating around in your grey matter. It’s time to bring the floating model to earth.
Approaching Modeling Risk
What does it mean to model risk well? In our book we say our goal is beating the competing model. If the competing model is arbitrary, inconsistent and mathematically ambiguous then our goals are humble. Alternatively, if our goal is absolute perfection when making any mathematical claim - then we will never get started.
That’s why we will start simply. Our goal is to build a model that uses well defined terms in a consistent manner that is mathematically unambiguous. Here is what I might start with in terms of things that matter. Several of these items will be decomposed further:
- Impact of SaaS Data Breach (loss in dollars)
- Value of SaaS Security Controls (likelihood of reducing breach)
- Budget (decision)
- Insurance Coverage (risk tolerance)
In the next article we are going to expand on this to build a quantitative model. In that model we will consider several SaaS third parties, varying amounts of data at each provider, strength of controls and a simple approach to defining risk tolerance. I will also expose you to some simple tools you can use to do this on your own.
If you feel that the NewCo example is farcical - you may need to get out more. And if you want the most up to date stats on the state of cloud providers consider reading RiskRecon’s Cloud Risk Surface Report.